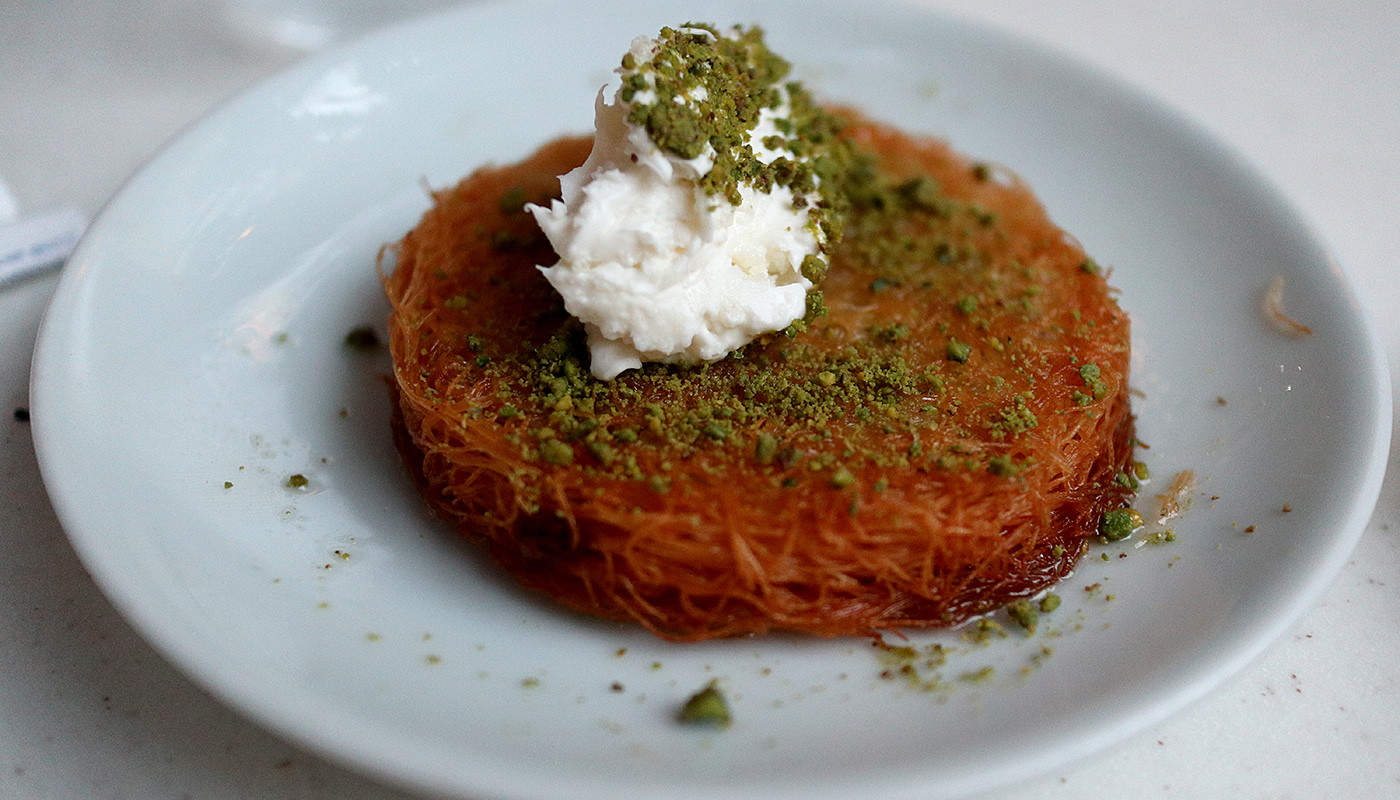
Often the most difficult thing in research in quant, just like every other area, is simply getting started. Once you know the question you’re trying to answer at least you have an idea of a potential path. Of course precisely which question you might want to ask to dependent on the objective. Whilst having a positive output to a question is often a preferred objective (wow, this trading strategy could make a lot money!), having a negative output is still useful information. Indeed, it could be helpful in the future (wow, I saved a lot of money because this trading idea is unlikely to work!).
If you’re in a fund, research needs to be gravitating towards a certain goal to make money, whether that’s generating P&L or saving money in some way, such as helping to publicise and market the fund. In academia, you’re a lot freer in the types of questions you ask. The objective is to generate a thought provoking publication, as opposed to P&L. If you’re doing some research in your spare time, you might have multiple objectives, including for example learning a new skill. The question we ask is also conditioned upon the resources we can employ in terms of people and time.
The question will also depend on the audience for its answer. Is it something for internal consumption in your firm? Is it for a presentation for a conference? Is the audience likely to be very technical, or should the output be more general? When it comes to deciding topics for example for presentations, I often take two approaches. One can be to use it as an opportunity to talk about my recent research or publications (particularly recently with The Book of Alternative Data). The other can be to try to help push you to do new research by placing deadline. If I know I need to do a presentation in a few months, there’s a deadline for me to work towards, and can help to focus the mind to get started.
In terms of specific topics for a presentation or research more broadly, at least in quant, there are a few areas I can think of (and this certainly isn’t an exhaustive list), at least to get the ball rolling.
Trading strategies and indicators
First off, perhaps the most obvious is around trading strategies and indicators to give us information about the market. It could be to explore a new alternative dataset, or to model something we have observed in markets, with the objective of creating an indicator and subsequently a trading signal. We might get ideas from a number of different places, whether it is from our own market observations, other research we read, Twitter etc.
Coding and automation
Another important area is examining new ways of coding or software engineering. Are there new libraries we could investigate to help us make our processes more efficient, when it comes to backtesting for example or number crunching data? Often these make for great demos in presentations, especially if you can combine it with interactive visualizations. I’ve found data science conferences as good starting points for learning about new Python libraries and also Twitter, as well as newsletters like Python Weekly.
Execution and TCA
Once your trading signals are done, there’s always room to see if you can improve your execution by using TCA. The larger the fund, the more imperative this will be.
Conclusion
It’s often difficult to find the right question to ask. However, asking the right question is a key part of the research process in quant and pretty much every other area. It is also important to note that even though lots of research ultimately won’t give us a positive outcome, a negative outcome often still gives us useful information.