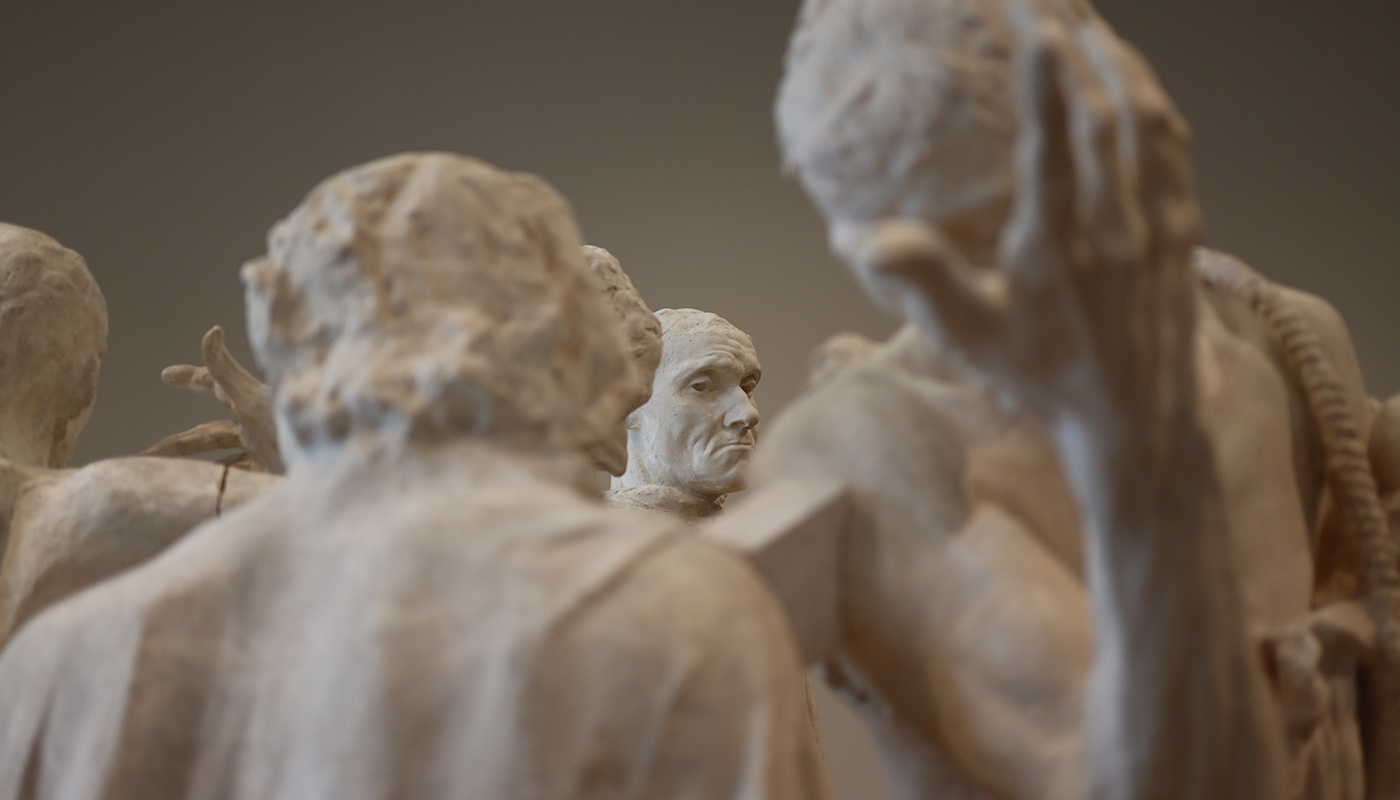
Price data is usually the first port of call for quants to use in trading strategies. There are many price based strategies which remain popular such as trend following. In general it tends to be easier to start with price data when building models. For one our signal and asset returns can be derived from the same dataset (albeit there can be some complexities when it comes to constructing total return indices).
If we’re trading macro assets like FX, should we stop at price data? As an advocate of using alternative dataset I would have to say no. The next logical dataset to look at it economic data. Of course I wouldn’t simply stop at that stage and continue to search for other dataset. After all economic data has its own issues, namely that it tends to be lagged and this has given rise to the use of alternative datasets to help proxy economic data on a higher frequency basis. It is difficult to term economic data as “alternative” given its been around for so long. However there are considerations which make economic data more complicated to deal with in general when compared to price data.
Frequency for economic data is generally lower, than price data, given most points tend to be monthly (or if we’re lucky weekly). This can make it more challenging in terms of data history. Point-in-time issues can be more difficult with economic data. Let’s say we have the US unemployment rate for Dec 2020. The data will itself will be released in Jan 2021. This will be only the actual release. This will be revised again. Each of these releases needs to be properly time stamped. If we are creating a trading strategy or forecast we need to be aware of this, to avoid look ahead bias.
Each headline data point will have many other granular components underneath it. For the US unemployment rate, you’ll have the unemployment rate for each state, for different industries and so on. So whilst the frequency is “low”, the number of potential columns can be very large. The data can also differ significantly between different countries, so it can be challenging to compare different countries.
All these complexities means that even before you can do any sort of analysis, there’s countless hours spent cleaning and aggregating the data (perhaps this isn’t too different from most data science problems!). However, once we have a nice clean dataset. Price data is obviously the key dataset for analysis of financial markets, but don’t discount other datasets such as economic data to shed further insights, even if it can be more challenging to deal with. And indeed I wouldn’t stop there, the next step is alternative data!