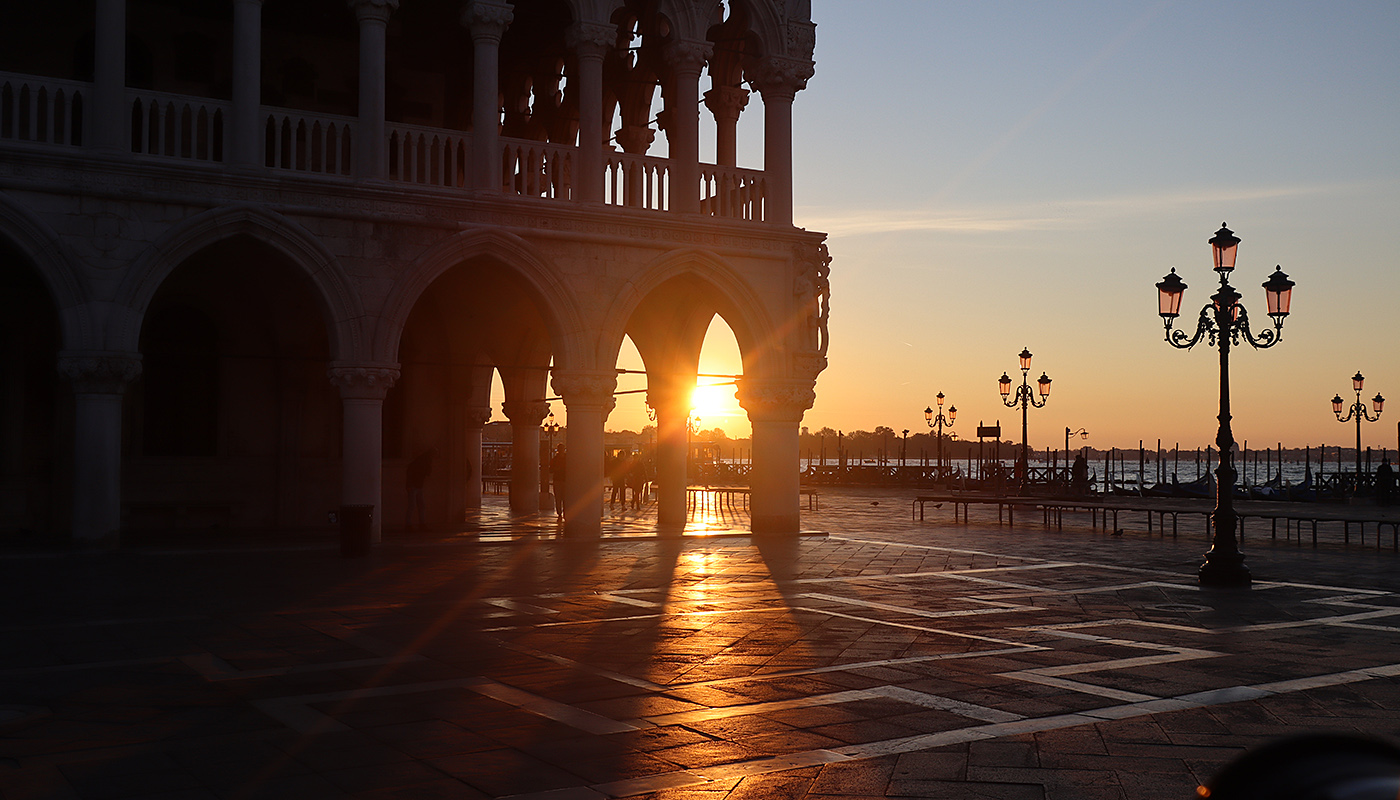
That burger you like. It looks cheap. But then you have to pay for the cheese. Then you have to pay for an onion ring. Then you have to pay for the chips. Want to upgrade to a “wagyu” patty (and no never do this), another way to extract a few further coins from you. By the end of the process, your cheap burger is a big expensive burger. It needs a knife and fork to cajole it into a biteable size. What seemed cheap, was not cheap, and you might have been better off just paying for a better burger to start, where you wouldn’t be subjected to repeated extras.
In finance, like in any business there are costs which seem to be incurred absolutely everywhere. I’m not talking about the obvious ones like the payroll and the office. Instead, I’m talking about those ever increasing subscriptions on stuff like software and data. Lots of software seems to be free like open source and indeed it is free. However, you still need software engineers to integrate it into your framework. You might need to pay support fees etc. Is it cheaper than traditional software? In many cases, yes, but it isn’t totally free, despite being open source.
When it comes to data, whilst many datasets are paid for (and can be very expensive), there are many free datasets around. Some are certainly worth investigating, and I think in general, there are quite a free sources of data which tend to get overlooked. At the same time, we need to assess, how “free” a dataset is before considering it.
If the data is of poor quality, with lots of gaps, and it requires a lot of cleaning, is it really “free”? We might actually be better off paying for a data vendor who supplies a similar dataset, where it is all normalized nicely and properly cleaned. This will save us the cost of cleaning and structuring the dataset ourselves.
There can be a tendency from folks like me (quants) to believe that we need to be involved throughout the whole of the data analysis process (and yes, I probably include myself in this category). Sure, we do need to be involved in the process, but there are stages in the process, where we can use other resources to speed up the process, whether it is software or data related.
Do we really need to write our own logger? Probably not, because it’s not the best use of our time, given that so many very good open source loggers. Do we really need to make sure that every dataset is free? Probably not again, because it’s likely to use up more of our time. These are only a few examples, but these questions will crop up again and again. If our time gets used up by stuff like this, we’ll have less time for places where we can really add value, in terms of coming of interesting hypothesises, and constructing trading models: which are ultimately the things we get paid for. Sometimes letting go is the most profitable way to use your time!