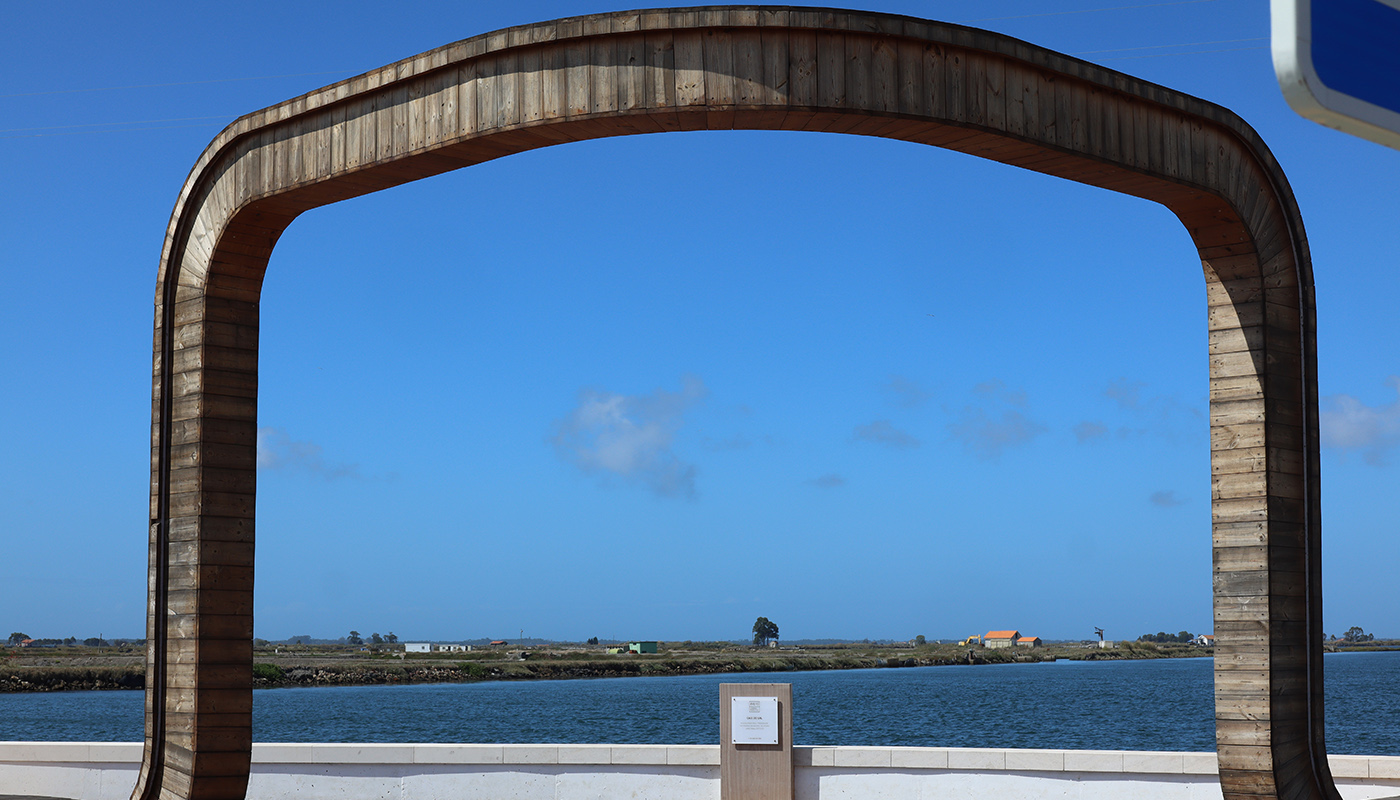
If we have no reference, we have no way to compare. If you’ve never had a burger before, there’s no point of reference for what a burger tastes like. Of course, there will be other points of reference, maybe a steak you’ve had before? Or if it’s a veggie burger, then perhaps tofu? It isn’t that our reference necessarily needs to be perfect. Our reference simply needs to be a method of comparison.
When it comes to financial markets, we use many models. How do we measure how good a model is? It really depends upon what the model in question is doing. Let’s say we have created a model to print some sort of exotic option. An obvious point of reference as a benchmark would be existing models, which are commonly in use, which we have for those same exotic options. Are the prices similar? If not, why? Perhaps the models we are using as benchmarks are have many more assumptions and hence, are not as realistic. In that situation, we would expect some sort of divergence, and being “close” to our benchmark may not be good. However, it is not only accuracy which might be of interest for a benchmark, in many cases, it will also be for example speed of calculation.
If we are creating a forecasting model, we also have this question of what is “good” in terms of model performance. It is something, Alexander Denev and myself had to think about at Turnleaf Analytics, a firm we have cofounded to do inflation forecasting. When creating our machine learning based models to forecast inflation, how do we judge their performance? Just as with our pricing model example earlier, we can use a benchmark. One benchmark could be to use a simple time series model for forecasting inflation, which is relatively straightforward to implement. Another approach can be to look at other inflation forecasts which are commonly cited within the market, such as those created by central banks and to model those, and this is one avenue we have looked at. Again the function of a benchmark is for comparison, and we want something that is representative, of the types of estimates in use.
Another approach is to rephrase our inflation forecasting question, as a trading strategy, and assess the performance of the trading strategy historically, without using a benchmark of other commonly used inflation forecasts. Having accurate inflation forecasts can provide inputs into many different types of trading strategies, such as those using inflation swaps and other rates instruments, as well as FX etc. If we have a trading strategy, our benchmark becomes a strategy which is commonly used by market participants (ie. the market beta). The historical trading strategy provides another way to test the performance of our forecasts.
In conclusion, a benchmark provides us a way to assess our own models. A benchmark needs to be representative of the types of models and approaches commonly used by market participants. If a benchmark is not accessible by market participants or could be constructed relatively quickly, then by definition, whatever measure we are using, is not really a benchmark. Instead, we might wish to consider it as a model in its own right. We can also rephrase our problem as a trading strategy. A trading strategy using our model forecasts provides another way of assessing the performance.