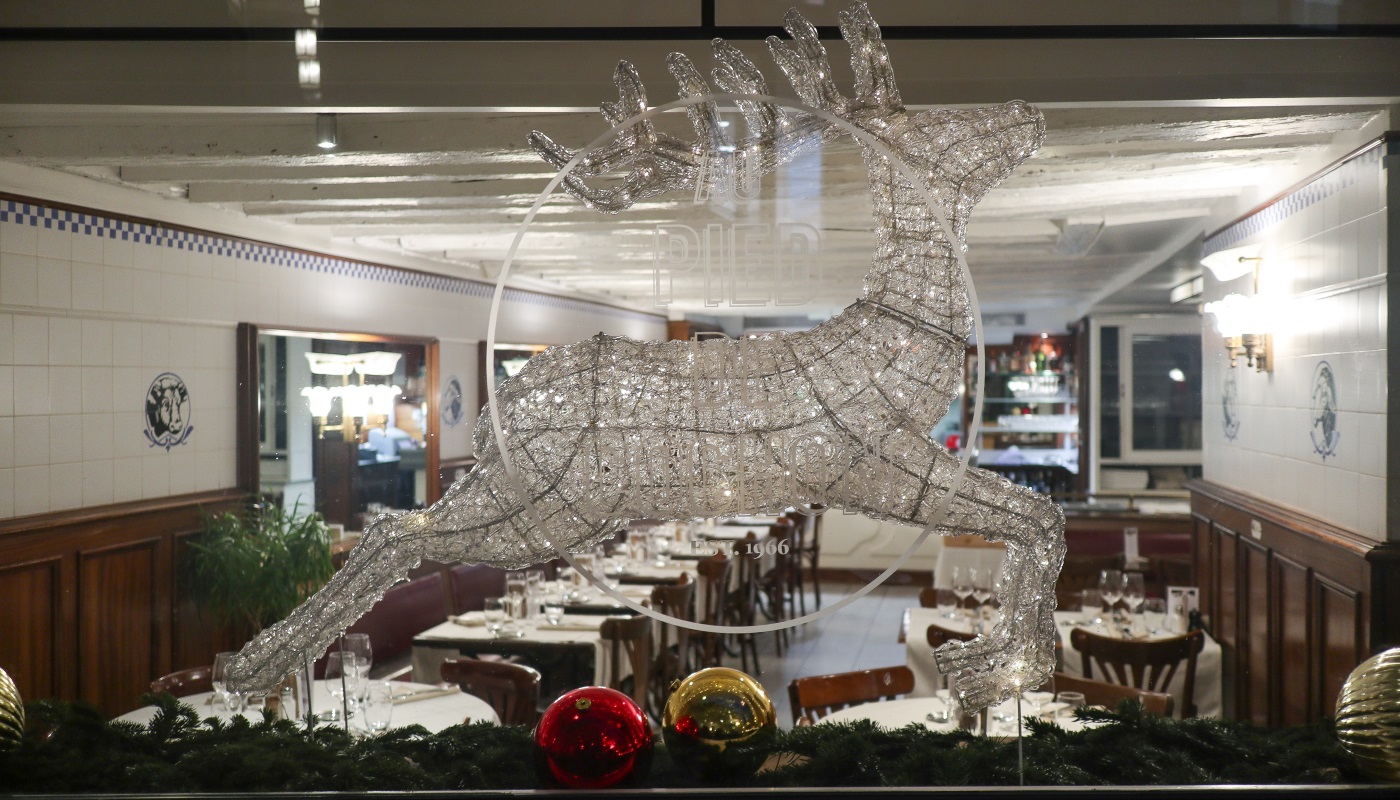
It’s impossible to know everything. Furthermore, as the cliche goes, the more you learn, the more painfully apparent it is, that there even more out there left to learn. I like burgers, but despite years of eating burgers, I’m not sure I can consider myself an expert. After all, I’ve only sampled a very small number of burgers available worldwide. I’m also pretty sure that eating large quantities of burgers, doesn’t make me an authority on how to make them. Consuming is somewhat easier than making them.
Finance is no different. You can’t know everything. The game keeps on changing too. Different themes come in and out of the market’s consciousness. New technology and the massive increase in alternative data, means we can run strategies which were inconceivable years ago.
Whilst you can’t know everything, there’s a lot which you still know! Each asset class has its own myriad of specific quirks which take years to learn. All this knowledge and expertise is valuable. So what can you do if you want to utilise all the domain knowledge you’ve acquired in finance, but also take advantage of all the new data and technology and future-proof what you?
The first thing to note, is that not everyone needs to suddenly be a systematic trader if they want to embrace the quant way of doing things. If you’ve been looking at markets from a fundamental perspective, quant tools can give you additional ways to analyse specific assets and give you additional insights. It’s about finding new alternative datasets to tell you something you didn’t already know (or quicker than traditionally available from other data sources). It’s about being to number crunch large quantities of data quickly using Python, rather than facing Excel spreadsheets which grind to a hold under the strain of the data.
Learning Python takes time. Whenever I teach my Python for finance course whether it’s to students or practitioners (taking bookings for 2020, if you’d like me to present my workshops in-house at your firm too!), one thing I stress is that coding is about practice. Attending lectures, reading books etc. is an important part of the process to get you started and to learn about new libraries etc. Ultimately, though this needs to be combined with spending time to code. Again, though, it doesn’t necessarily mean you suddenly need to become an expert in Python to start doing some interesting analysis. Solving smaller problems on the way, will help encourage you to learn more Python.
However, learning how to use quant tools doesn’t mean you should forget what you already know. It’s the combination of financial domain knowledge with quant tools, which is what will help to distinguish what you do, rather than just replacing one for the other. That’s the best way to future-proof your investment process.