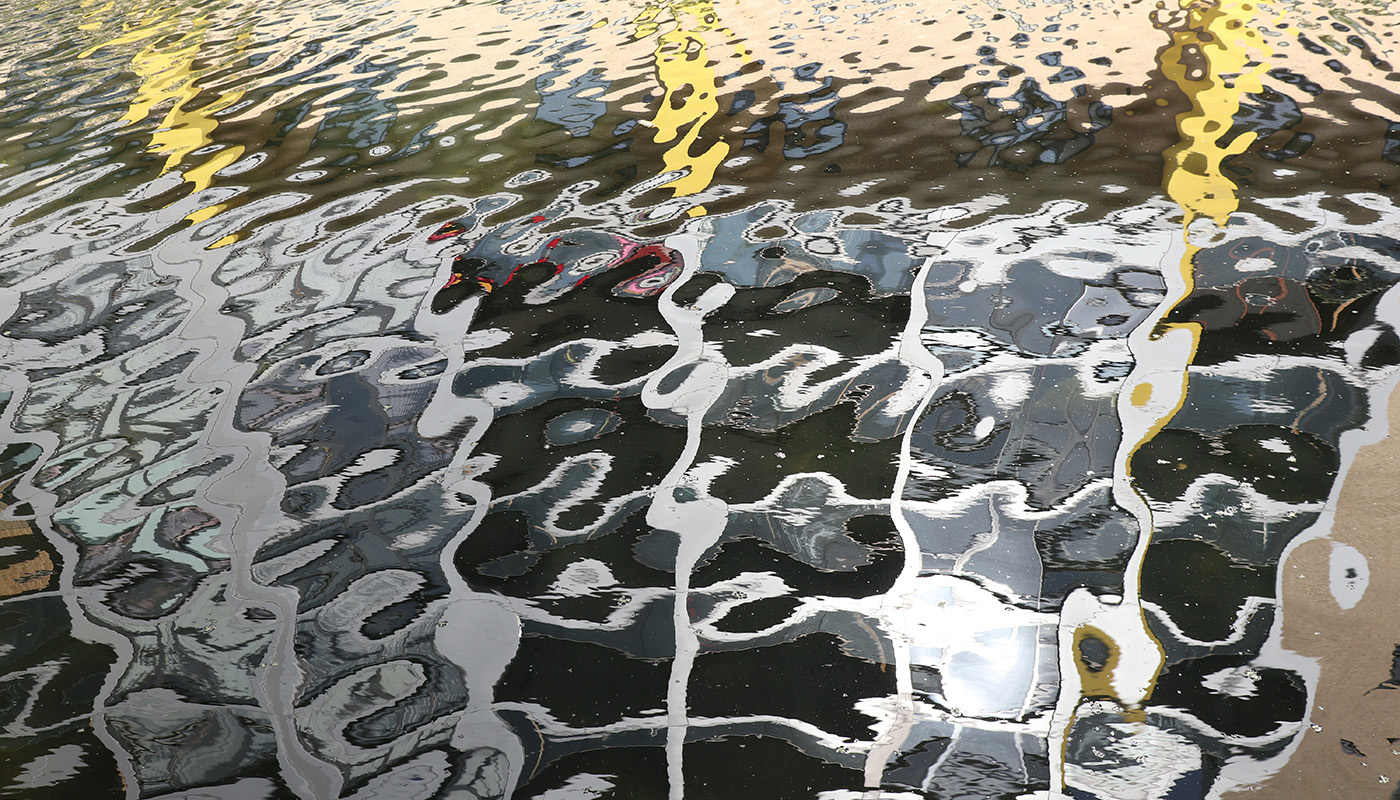
Something’s been missing the past few months. It’s been pretty easy for me to identify that very specific missing element… burgers. I have made my own burgers at home, and they were pretty good. Yet, there were still something missing. It’s not quite the same as the ones you get at Burger & Lobster, and I’m just going to forget about replicating those triple cooked chips at Goodman. No burger I’ve made has given me that hearty feeling I get from Meat Liquor (Although I have to admit homemade bread buns are probably better than the usual burger buns you get at all these restaurants).
However, at other times, trying to identify what’s missing is somewhat difficult and it isn’t as clear cut. At one of the last Thalesians’ talks to be conducted in person, before the coronavirus crisis truly took hold in the UK, Prof David Hand was speaking (although we have organised many webinars subsequently which you can attend). He introduced the concept of dark data, which is essentially data you don’t know about. To echo the words of Donald Rumsfeld, these are unknown unknowns.
When it comes to trading strategies, we develop models that are approximations of the reality of the market. We choose specific datasets or factors to incorporate into a model. Trying to incorporate every nuance of the market into a single model isn’t feasible. Furthermore making a model excessively complex to try to incorporate everything is going to be difficult to interpret. Trying to run multiple models, each of which capture different types of market behaviour, can often be easier to interpret. We can also separate out some of the complexity this way, and also have weighting schemes to allocate risk between models.
For datasets, obviously market data is a key part of most trading models, as is economic data. However, that leaves a lot of other datasets, less commonly used datasets that we could look at, which come under the umbrella of alternative data. If you’d like to know more about that subject, I recommend reading The Book of Alternative Data, written by Alexander Denev and I, which is on pre-order at Amazon and should be out in a few weeks (including on Kindle). The key point is about combining various datasets together to help augment existing ones, in an effort to find that elusive missing data.
That missing data might be derived from satellite images, it could be from news data etc. Often it can be obvious what’s missing, but is difficult to measure, such as political risk. This can be difficult to incorporate into models, although there are new datasets that can help (I recently wrote a paper on Thorfinn’s political datasets for example).
There are also many challenges more broadly with alternative data and we go over these at length in the book, ranging from specific technical issues like dealing with missing data points to structuring data which isn’t originally numerical (such as text). More broadly we note that to use alternative data effectively, you need to have the right folks hired, and a willingness to use such datasets.
It’s difficult to capture every possible factor or behaviour, but at the same it doesn’t mean that we can’t improve our trading models. Alternative data can help us plug some of the missing gaps we might find.