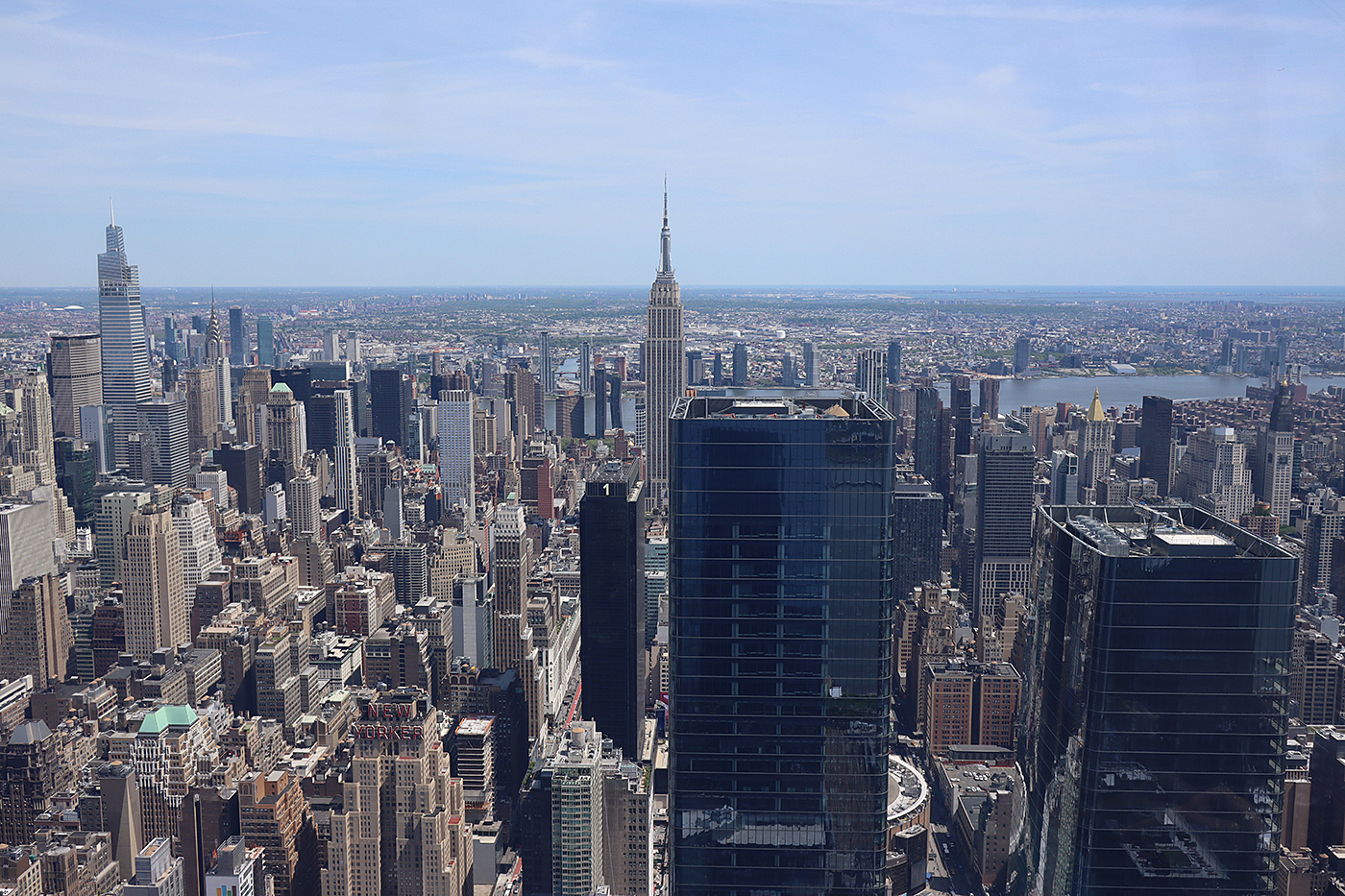
I like burgers and I also like to try new burger joints. There’s always a risk to trying new a place. Maybe the burger won’t be cooked in the same way, maybe the patty won’t be quite as good quality, maybe the chips will be soggy etc. In some cases, it could be a factor that I’ve never thought of, which impacts a burger. Basically, making a burger is easy, but making a great burger is more challenging.
Collecting and forecasting economic data is also extremely challenging, like making the perfect burger, and there are many factors that need to be considered when doing do. There has been a lot of chatter on Twitter recently, such as from @balajis, on the subject of payroll data, where he suggests that recent economic data on the labour market published by the BLS is not entirely truthful. Some reasons he cites includes the fact that during the last 14 months, the change in non-farm payrolls (ie. NFP) has surprised the consensus to the topside (source @bespokeeconomics) and also the discrepancy between NFP (gathered from the Establishment Survey) and the Household Survey, from which is published statistics including the unemployment rate.
If we purely look at the discrepancy between the Household Survey and the Establishment Survey, @bdonnelly notes that historically the Household Survey has generally been very volatile on a month to month basis, even more so than the Establishment Survey (which is noisy if we were to look at it in isolation), and is one reason why the market is so focused on the Establishment Survey.
Moreover, collecting economic data is extremely challenging. You could argue the fact that the NFP data is frequently revised by the BLS is a reflection of this. When collecting economic data, there are all sorts of assumptions that are made, and whilst these can be challenged (such as by @ModeledBehaviour), the key point is that these are transparent (and indeed many are published by the BLS on their website, such as the impact of the Birth Death model which they use when compiling the Current Employment Statistics). Given how much markets move on the release of the NFP number, it does suggest that market participants who are staking large amounts of capital on the number (ie. those with “skin in the game”), they believe the number is worth following.
At Turnleaf Analytics, the firm I cofounded with Alexander Denev, to do economic forecasts using machine learning and alt data, one thing we’ve learnt is that forecasting economic number is extremely challenging. Whilst we have started with forecasting inflation, we are now forecasting unemployment and soon also NFP. Creating a forecast that can beat consensus has required a lot of work (and data), and isn’t something you can accomplish with a simple model.
There can be all sorts of reasons why the market consensus has been underestimating NFP compared to the actual prints in recent months. From a forecasting perspective (and hence trading!), if consensus gets it wrong, it is also an opportunity. We need to first consider the metric for what is a “good” forecast. The direction of the surprises is important, but we should also measure the performance of a forecast by other metrics such as the mean absolute error. Indeed, if we look at the mean absolute error of the consensus vs. actual on NFP (3M SMA to smooth it), it has been smaller over the past year, than the year before that. The direction of the surprise matters, but so does the magnitude, when we consider the market reaction.
Another way to assess the utility of the forecast is whether it can be monetised by a trading rule. We have done a lot of research on our inflation forecasts showing how it can be used to trade FX systematically for example (and are exploring other asset classes).
It is also possible for a economic forecast model to continually undershoot or overshoot a number, because of assumptions made in the modelling process, such as missing a factor, a lack of data to capture certain dynamics, a change in the regime, overfitting etc. In our economic forecasting, we are continually monitoring all these moving parts such as the model we are using, whether additional data can help etc. Modelling economic data well requires a continually assessment of the model performance and research to see whether there are ways to improve this.
Forecasting economic data isn’t easy, neither is collecting it, in particular for series such as NFP, which is frequently revised. However, the difficulty in doing both tasks does not reduce the utility of both tasks. Indeed, the fact that consensus often gets it wrong, means there can be opportunities in trying to beat consensus.